Introduction
Satellite imagery has revolutionized how we monitor and analyze our planet, and its applications in agriculture are no exception. With the help of artificial intelligence (AI), satellite photos may be converted into useful crop data that gives farmers important information about their crops’ health and prospective production.
Through advanced AI algorithms, satellite images can be analyzed to identify patterns, detect anomalies, and extract valuable crop-related information. Integrating multiple data sources, such as weather data and historical records, further enhances the accuracy and reliability of the insights generated. By harnessing the power of AI, farmers can make informed decisions about irrigation, fertilization, pest control, and overall crop management, leading to optimized yields and reduced environmental impact.
From pixels to insights, AI-driven crop monitoring represents a significant leap forward in sustainable and efficient agricultural practices. By leveraging the power of satellite imagery and advanced algorithms, farmers can optimize their resources, improve crop health, and contribute to the global challenge of ensuring food security for a growing population. Let us embark on a journey into AI and satellite imagery, where pixels become actionable crop data, enabling farmers to make data-driven decisions for a better future in agriculture.
The Role of AI in Agricultural Innovation:
AI is pivotal in revolutionizing the agricultural industry by offering innovative solutions to improve productivity, sustainability, and efficiency. It enables precision farming techniques by analyzing data and providing real-time insights for precise planting, irrigation, fertilization, and pest control. AI-powered imaging systems and computer vision algorithms can monitor crops, detect diseases, and identify nutrient deficiencies. Intelligent robots equipped with AI algorithms perform tasks such as harvesting and weed control with precision. AI also optimizes the agricultural supply chain, enables predictive analytics for decision-making, and promotes sustainable farming practices. The integration of AI in agriculture is transforming how we produce food and addressing global challenges such as food security and climate change. Embracing AI in agricultural innovation paves the way for a more sustainable and productive future in farming.
Understanding Satellite Imagery: Pixels and Beyond
Satellite imagery plays a crucial role in providing valuable data for agricultural applications. By capturing images of Earth’s surface from space, satellites give information on crops, soil conditions, and environmental factors. However, interpreting these images can be challenging due to the vast amount of data involved. This is where AI comes in.
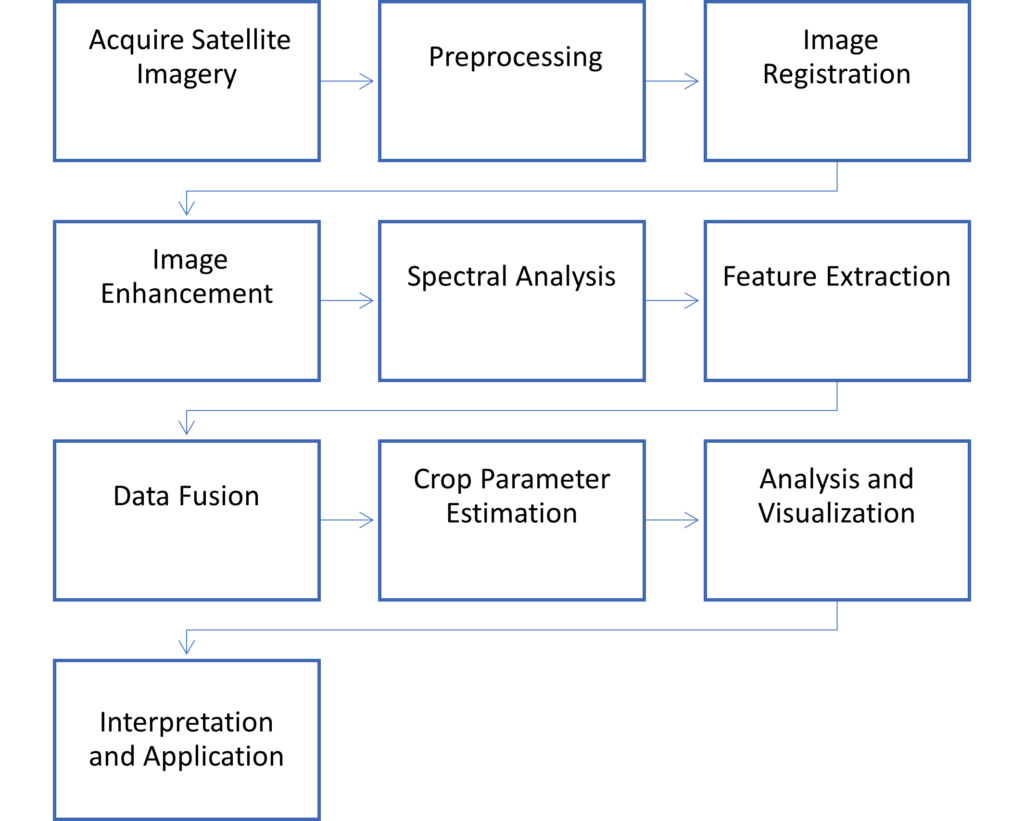
AI algorithms can analyze satellite images at a scale and extract meaningful insights. They can identify and classify different types of crops, detect patterns and anomalies, assess vegetation health, and estimate crop yield potential. By combining satellite imagery with AI, farmers and agricultural experts can make informed decisions regarding planting, irrigation, fertilization, and pest management.
Beyond pixels, AI can go further by integrating other data sources, such as weather data, soil moisture levels, and historical records. This holistic approach enhances the accuracy and reliability of the insights provided by satellite imagery, enabling farmers to optimize their practices and maximize crop productivity.
In agriculture, AI is unlocking the potential of satellite imagery, transforming raw pixels into actionable crop data that empowers farmers to make data-driven decisions and optimize their farming operations for improved efficiency and sustainability.
AI Algorithms for Image Analysis: Unveiling the Invisible
In agriculture, the power of AI algorithms for image analysis is revolutionizing how we perceive and understand the invisible aspects of crop health and productivity. By harnessing the capabilities of artificial intelligence, farmers and agricultural experts can uncover valuable insights from images captured by satellites, drones, and other imaging technologies.
AI algorithms are designed to analyze vast amounts of image data, extracting valuable information beyond what the human eye can perceive. These algorithms can detect subtle variations in vegetation health, identify crop diseases and pests, assess nutrient deficiencies, and even accurately predict crop yields.
One of the critical advantages of AI algorithms is their ability to learn and adapt to large datasets. By training on diverse images, these algorithms become increasingly proficient at recognizing patterns and anomalies, enabling them to provide more accurate and reliable insights over time.
The application of AI algorithms for image analysis is not limited to satellite imagery alone. It extends to other imaging technologies, such as drones with high-resolution cameras or multispectral sensors. These tools enable farmers to capture detailed and up-to-date information about their crops, allowing for timely interventions and precise decision-making.
With AI algorithms, the invisible becomes visible. By unveiling the intricate details hidden within images, these algorithms empower farmers to proactively address crop health issues, optimize resource allocation, and ultimately enhance agricultural productivity sustainably.
There are several AI algorithms used for image analysis in agriculture. Some of the commonly employed algorithms include:
- Convolutional Neural Networks (CNN): CNNs are widely used for image classification and object detection tasks. They are trained on large datasets and can automatically learn and extract features from images, enabling them to classify and detect objects of interest in agricultural photos accurately.
- Support Vector Machines (SVM): SVM is a popular algorithm for image classification and regression tasks. It works by finding an optimal hyperplane that separates different classes in the image data, allowing for accurate classification of crops, diseases, pests, and other objects in agricultural images.
- Random Forest: This ensemble learning algorithm combines multiple decision trees to make predictions. It can be used in agriculture for crop classification, disease identification, and yield estimation based on image data.
- Deep Learning Models: Deep learning models, such as recurrent neural networks (RNNs), long short-term memory (LSTM) networks, and generative adversarial networks (GANs), are increasingly being used for image analysis in agriculture. These models can capture complex patterns and relationships in agricultural images, enabling image segmentation, anomaly detection, and crop growth prediction tasks.
- K-means Clustering: K-means clustering is a popular unsupervised learning algorithm used for image segmentation in agriculture. It groups similar pixels based on their color, texture, or other features, allowing for the identification of different regions or objects within an image.
The above are a few examples of the AI algorithms used in image analysis for agriculture. However, the choice of algorithm depends on the specific task, dataset, and available resources, and researchers and practitioners continue to explore and develop new algorithms to improve the accuracy and efficiency of image analysis in agriculture.
Data Fusion and Integration: Combining Forces for Comprehensive Insights
In agricultural data analysis, data fusion and integration are pivotal in providing comprehensive insights into crop health and productivity. By combining multiple data sources, such as satellite imagery, weather data, soil composition data, and crop sensor data, farmers and agricultural experts can better understand their fields and make informed decisions.
Data fusion involves merging different data sets to create a unified and cohesive representation of the agricultural landscape. This process enables the integration of diverse data types, allowing for a more comprehensive analysis and interpretation of the information. By combining data from various sources, farmers can obtain a more accurate and complete picture of the factors influencing crop growth and yield.
Farmers can detect patterns, identify trends, and make data-driven decisions to optimize their farming practices by integrating data from multiple sources. Conversely, integration involves seamlessly blending different data streams into a unified platform or system. This integration enables real-time monitoring and analysis, facilitating timely interventions and proactive decision-making.
Advanced technologies, including machine learning, big data analytics, and cloud computing, power the fusion and integration of agricultural data. These technologies enable the processing, analyzing, and storing vast amounts of data, ensuring farmers have access to timely and actionable insights.
Data fusion techniques used in satellite imagery to process crop data involve integrating and combining data from various sources to derive accurate and meaningful crop information. Here are some commonly used data fusion techniques in this context:
- Spectral Fusion: Spectral fusion combines spectral information from satellite images with different spectral bands. Merging these bands enhances the spectral resolution and provides a more detailed representation of the crops. Spectral fusion helps identify specific crop characteristics such as vegetation health, stress levels, and disease outbreaks.
- Spatial Fusion: Spatial fusion involves merging satellite images captured at different resolutions. It allows for integrating high-resolution photos that capture fine details with low-resolution images that cover larger areas. Spatial fusion helps obtain a comprehensive view of crop conditions by combining detailed and broader-scale information.
- Temporal Fusion: Temporal fusion integrates satellite images acquired at different time points to analyze crop development and changes over time. Comparing and combining images from multiple dates enables the detection of crop growth patterns, phenological stages, and temporal changes in vegetation health. Temporal fusion is valuable for monitoring crop progress, detecting anomalies, and predicting yield potential.
- Data Assimilation: Data assimilation combines satellite imagery with ground-based data, such as weather data, soil measurements, and crop sensor data. It assimilates these diverse datasets into crop growth models or algorithms to improve the accuracy of crop monitoring and prediction. Data assimilation allows for a better understanding of crop responses to environmental factors and enhances the precision of crop management decisions.
- Feature Extraction and Classification: Feature extraction techniques identify and extract specific features from satellite imagery that are relevant to crop monitoring, such as vegetation indices, texture patterns, or shape characteristics. These extracted features are then used for classification purposes, where machine learning algorithms categorize different land cover classes (e.g., crops, weeds, soil) within the imagery. Feature extraction and classification techniques enable precise mapping and monitoring of crops at a large scale.
Using these data fusion techniques, satellite imagery can extract crop-related information, monitor crop health and development, detect anomalies, and support agricultural decision-making. These techniques enhance crop data’s accuracy, spatial resolution, and temporal coverage, ultimately improving crop management and productivity.
Empowering Farmers: Actionable Insights from Satellite Images
Satellite images have revolutionized the way farmers monitor and manage their crops. With the advent of advanced artificial intelligence (AI) technologies, these images are now transformed into actionable insights, providing farmers with valuable information about their fields, crop health, and yield potential.
Using AI algorithms, satellite images can be analyzed in real-time, extracting valuable data and identifying patterns that are invisible to the naked eye. These algorithms leverage machine learning techniques to detect and classify crop features, such as plant density, growth stage, and stress indicators. By analyzing the pixel-level information in satellite images, AI can identify areas of concern and provide targeted recommendations for intervention.
Farmers can make informed judgments and take proactive steps to improve their farming practices thanks to the valuable insights from satellite photos. They can detect early signs of crop diseases, nutrient deficiencies, or water stress, allowing timely interventions to prevent yield loss. Additionally, farmers can assess crop performance across different areas of their fields, enabling precision management and resource allocation.
The power of satellite imagery lies in its ability to capture large-scale and high-resolution data, providing a bird’s-eye view of agricultural landscapes. This enables farmers to monitor their crops at a macro level and identify trends and patterns that may impact productivity. By combining historical satellite data with real-time observations, farmers can gain a deeper understanding of long-term trends, climate patterns, and the effects of management practices on crop performance.
Farmers can make data-driven decisions, optimize resource allocation, and implement precision agriculture techniques with access to actionable insights from satellite images. This enhances productivity and contributes to sustainable farming practices by minimizing input and reducing environmental impact.
In conclusion, transforming satellite images into actionable insights through AI technologies has revolutionized how farmers monitor and manage their crops. Farmers may enhance their decision-making, increase crop yields, and contribute to a more sustainable and effective agricultural system by utilizing the power of these insights.
Advantages and Challenges of AI-driven Crop Monitoring
AI-driven crop monitoring offers numerous advantages in enhancing agricultural practices but also comes with challenges. Understanding the benefits and limitations is crucial for farmers and stakeholders implementing AI-based solutions.
Accurate and Timely Insights: AI algorithms can analyze vast satellite imagery data and provide precise and timely insights into crop health, disease detection, and yield potential. This allows farmers to address issues and optimize their farming practices proactively.
Cost-effective Solution: AI-driven crop monitoring eliminates the need for manual and labor-intensive field inspections. By leveraging satellite imagery and AI algorithms, farmers can monitor large land areas efficiently and at a lower cost than traditional methods.
Increased Efficiency and Productivity: With AI-powered crop monitoring, farmers can identify specific areas of their fields that require attention. Farmer setting interventions such as irrigation, fertilization, or pest control, farmers can optimize resource allocation and maximize crop productivity.
Sustainability and Environmental Benefits: AI-driven crop monitoring enables precision agriculture, allowing for the precise application of water, fertilizers, and pesticides. This targeted approach minimizes waste, reduces environmental impact, and promotes sustainable farming practices.
The Future of AI in Agriculture: Driving Sustainable Food Production
Artificial intelligence (AI) is swiftly transforming the agricultural sector, revolutionizing how we cultivate and produce food. Due to its ability to evaluate massive amounts of data, maximize resource allocation, and enhance decision-making, artificial intelligence (AI) can increase sustainable food production and address the issues connected with feeding an increasing number of people worldwide.
AI-powered technologies, such as machine learning algorithms, remote sensing, and robotics, are being utilized to improve farming practices and increase efficiency. This article explores the future of AI in agriculture and its impact on sustainable food production. It delves into various areas where AI is applied, including crop monitoring, yield prediction, pest and disease management, irrigation optimization, and precision farming.
By leveraging AI, farmers can make data-driven decisions, optimize the use of resources, and reduce environmental impact. AI-enabled crop monitoring allows for the early detection of diseases, pests, and nutrient deficiencies, enabling proactive intervention and minimizing yield losses. Precision farming techniques, guided by AI algorithms, allow the targeted application of fertilizers, pesticides, and water, reducing waste and improving sustainability.
Moreover, AI-driven predictive models help farmers anticipate yield outcomes, optimize harvest timings, and plan logistics effectively. This contributes to better market forecasting, reduced food waste, and improved supply chain management. AI-powered robots and automation systems are also transforming labor-intensive tasks such as planting, harvesting, and sorting, increasing productivity and reducing dependency on manual labor.
However, challenges such as data privacy, technological infrastructure, and equitable access to AI solutions must be addressed as AI advances in agriculture. Collaborative efforts between technology developers, farmers, policymakers, and researchers are essential to ensure AI’s responsible and inclusive agricultural implementation.
The future of AI in agriculture holds immense potential to drive sustainable food production, enhance resource efficiency, and mitigate the environmental impact of farming practices. We can develop a more resilient and sustainable food system that can meet the needs of a growing global population while leaving the minor possible ecological imprint by utilizing AI.
Case Studies: Real-World Applications of AI in Crop Monitoring
In the world of agriculture, the adoption of AI technologies for crop monitoring has proven to be a game-changer. Farmers can gain valuable insights into their crops’ health, growth, and yield potential by harnessing AI algorithms and satellite imagery.
Case Study 1: Optimizing Pest Management In a large-scale citrus orchard, AI-based crop monitoring systems were deployed to detect early signs of pest infestation. By analyzing high-resolution satellite images, AI algorithms could identify specific areas affected by pests, enabling targeted intervention. This resulted in a significant reduction in pesticide usage, minimized crop damage, and improved overall yield.
Case Study 2: Precision Irrigation In a water-stressed region, AI-driven crop monitoring techniques were employed to optimize irrigation practices. Satellite images were analyzed to determine the moisture levels in the soil, crop water requirements, and areas prone to water stress. This information was used to implement precision irrigation strategies to ensure water resources were utilized efficiently and minimize water wastage.
Case Study 3: Yield Prediction and Quality Assessment Using AI algorithms, a large-scale soybean farm implemented crop monitoring systems to predict yield and assess crop quality. By analyzing satellite images throughout the growing season, AI models could accurately estimate crop yield, identify areas of potential underperformance, and provide recommendations for optimizing inputs such as fertilizers and growth regulators. This resulted in improved yield outcomes and enhanced profitability.
Case Study 4: Disease Detection and Management AI-powered crop monitoring systems have been instrumental in the early detection and management of plant diseases. Farmers can identify hotspots, track disease progression, and implement targeted control measures by analyzing satellite images and utilizing machine learning algorithms. This has reduced crop losses, improved disease management, and increased productivity.
These real-world case studies demonstrate the tangible benefits of AI in crop monitoring. Farmers can make informed decisions, optimize resource allocation, and mitigate risks by harnessing AI algorithms and satellite imagery. AI-driven crop monitoring enables proactive intervention, reduces input wastage, improves yield outcomes, and contributes to sustainable agricultural practices. As AI continues to advance, the potential for its application in crop monitoring is vast, promising a more efficient, productive, and sustainable future for agriculture.
Overcoming Challenges: Improving Data Quality and Algorithm Accuracy
Despite the enormous potential of AI-driven crop monitoring, several issues must be resolved to guarantee the precision and dependability of the conclusions drawn.
- Data Quality Assurance, One of the critical challenges in AI-based crop monitoring is ensuring the quality and reliability of the input data, particularly satellite imagery. Issues such as cloud cover, sensor limitations, and variations in image resolution can affect data accuracy. To overcome this challenge, advanced image processing techniques, including data fusion and image enhancement, are employed to improve the quality of satellite images. Additionally, ground truth data collected through on-site measurements and field observations can be used to validate and calibrate the satellite data.
- Algorithm Development and Calibration Developing accurate and reliable AI algorithms for crop monitoring requires robust training data and rigorous calibration. Training data must be diverse and representative, including various crop types, growth stages, and environmental conditions. Furthermore, algorithms must be continuously updated and refined based on real-world performance feedback. This iterative algorithm development and calibration process ensures that the AI models become more accurate and effective over time.
- Integration of Multiple Data Sources To enhance the accuracy and completeness of crop monitoring insights, integrating multiple data sources is crucial. This includes satellite imagery and ground-based data such as weather records, soil moisture sensors, and crop phenology observations. By combining and analyzing data from various sources, AI algorithms can generate more comprehensive and reliable insights for farmers.
- Field-Specific Adaptation Agricultural landscapes can vary significantly from one location to another, with variations in crop types, farming practices, and environmental conditions. To ensure the relevance and accuracy of AI-driven crop monitoring, algorithms must be adapted and customized for specific regions and crops. This involves considering local knowledge, fine-tuning algorithms based on regional data, and accounting for unique agronomic practices and challenges.
Addressing these challenges and continuously improving data quality and algorithm accuracy can enhance the effectiveness of AI-driven crop monitoring. This, in turn, empowers farmers with reliable and actionable insights to make informed decisions, optimize resource allocation, and improve overall crop management practices.
Conclusion
The transformational potential of AI in agriculture is evident in its ability to convert satellite images into actionable crop data. Farmers can now harness the power of satellite imagery to gain valuable insights into crop health, yield potential, and environmental conditions by integrating AI algorithms, data fusion techniques, and advanced image analysis. This article has explored the various aspects of AI-driven crop monitoring, including the role of AI in agricultural innovation, the study of satellite imagery, AI algorithms for image analysis, data fusion, and integration, and the advantages and challenges of AI-driven crop monitoring.
The benefits of AI in agriculture are substantial. Farmers can use it to make data-driven decisions, allocate resources more efficiently, increase crop output, and have a minor negative environmental impact. AI-driven crop monitoring also enhances the ability to detect and respond to crop diseases, pests, and other stressors, leading to more effective and timely interventions. Furthermore, AI empowers farmers with precise and actionable insights, enabling them to achieve sustainable food production and contribute to global food security.
However, challenges remain in the implementation of AI in agriculture. These challenges include data quality, algorithm accuracy, technical infrastructure, and the need for skilled personnel. Overcoming these challenges requires concerted efforts from researchers, industry players, and policymakers to improve data collection and quality, refine AI algorithms, develop user-friendly platforms, and ensure accessibility to AI technologies for all farmers.
The future of AI in agriculture is promising. Ongoing collaborations and research initiatives are driving advancements in AI technologies while industry players continue to invest in AI-driven solutions. The development of international research networks facilitates knowledge exchange and standardization, further accelerating the adoption and effectiveness of AI in agriculture. In conclusion, artificial intelligence can transform crop monitoring and advance environmentally friendly agriculture methods. By leveraging the power of satellite imagery and AI algorithms, farmers can gain valuable insights and make informed decisions to optimize their farming operations. AI will be essential in influencing the future of agriculture through continuing research, innovation, and cooperation. Thanks to AI, farmers can manage the difficulties of feeding an expanding population while preserving the environment.